The project will entail data processing and data analysis of behavioral and biomechanical data (collected) from experiments. The goal will be to develop a manuscript for publication at the end of the Spring semester 2021
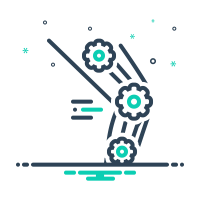
The project will entail data processing and data analysis of behavioral and biomechanical data (collected) from experiments. The goal will be to develop a manuscript for publication at the end of the Spring semester 2021
We are developing machine learning (ML) methods to understand how people influence each others’ behavior in social networks. For example, on Twitter, do users influence the content shared or posted by their followers? Methods that can identify such patterns of influence will play a role in studying, e.g., the spread of misinformation on social media sites.
The goal of the project is twofold: 1) to better understand and further improve the use of low cost air pollution sensors and 2) to analyze and characterize air pollution data in sub-Saharan Africa. Air pollution kills an estimated 700,000 people per year in Africa, but existing air pollution data in Africa is extremely sparse and estimates of the associated mortality are uncertain. Low cost air pollution sensors have the potential to rapidly revolutionize air quality awareness and data availability in data-sparse areas of the world, including sub-Saharan Africa. However, use of low cost sensors requires careful calibration, performance evaluation, and other quality assurance before the data can be fully trusted to the same degree as regulatory-grade monitors. As part of a larger project led by Dr. Westervelt, fine particulate matter (PM2.5) sensors have already been deployed in several African megacities, including Kinshasa, Democratic Republic of Congo; Nairobi, Kenya; Kampala, Uganda; Accra, Ghana, and Lomé, Togo. In Kampala and Accra, sensors are co-located with a regulatory-grade PM2.5 instrument for several months, allowing for a direct comparison between low cost and regulatory-grade PM2.5 measurements, and also allowing for the development of calibration factors.
The spread of COVID-19 has led to unprecedented and ongoing changes to daily life, including shelter-in-place orders, widespread closing of businesses and schools, and work-from-home and school-from-home at previously unknown levels. These changes in behavior are placing extraordinary demands on the Internet. This project will measure the Internet’s ability to meet these demands, including comparing its performance before, during, and after the peak of COVID-19; whether the amount of change varies between areas heavily impacted by COVID-19 and those less impacted; and whether and how large networks adapt. To provide this rich understanding, this project will combine multiple Internet-scale datasets that provide complementary views to investigate how responses to COVID-19 have impacted the Internet and how networks have reacted. Measuring the network impact of COVID-19 will illuminate the Internet’s strengths and weak points and is a crucial step towards improving the Internet’s future resilience in the face of pandemics, natural disasters, large scale conflict, and terrorist attacks.
The main goal of this work is to assess if storms have increased in frequency over Antarctica. It is theorized that climate change will increase the intensity of the winds and frequency of the storms. With ICESat 2 satellite laser altimetry, we can count the number of storms and blowing snow events. ICESat 2 is a photon counting laser and generates terrabytes of data each day. Innovative data science techniques are needed to handle the data and analyze it. This project is, therefore, a suitable topic for a masters student that combines an important problem in Geophysics and climate science with a great Data Science application.