The federal government spends billions of dollars a year supporting rural broadband (internet access), subsidizing build-out in low-density areas that do not have broadband (unserved areas). However, it is not clear whether the rural areas most in need are receiving a fair share of the funding. Using a very large dataset of broadband availability, census data and recent auction results, the project will analyze whether unserved areas with high racial diversity or lower median income are receiving a fair share of funding. Depending on team size, we will also attempt to create a shareable master data set building on OpenStreetMap and other sources that provides key data points for census units.
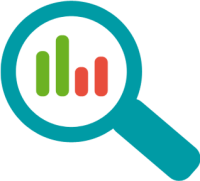