Immune checkpoint blockade therapy has shown successful clinical outcomes in the treatment of various solid tumors such as head and neck squamous cell carcinoma (HNSCC), melanoma, non-small cell lung cancer (NSCLC) and others. However, immune checkpoint inhibitors work best in patients who exhibit certain tumor biomarkers. In a collaboration with the Department of Hematology Oncology, the Department of Systems Biology, and the Mailman School of Public Health at Columbia University we aim to identify biomarkers which are associated with treatment outcome in patients with solid tumors who underwent immunotherapy. The project includes bioinformatic analysis of sequencing data. Mentoring and training will be provided.
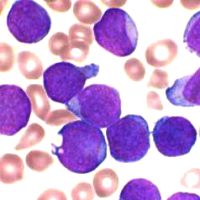