Traditionally, these types of data are routinely neglected in hand-crafted constitutive models due to the complexity. Instead, descriptors such as void fraction, dislocation density, and other statistical measures of the microstructures are often incorporated into yield surface or hardening rules (e.g. Gurson damage model, critical state plasticity). In this work, we will overcome this technical barrier by using a deep convolutional neural network to deduce low-dimensional descriptors that best describes the physics of the deformation process of polycrystals. With deep Q reinforcement learning to automate the trial-and-error process, we may explore the decision tree with a large number of trials that are impossible to be done manually. This treatment will empower us to discover the underlying mechanics of polycrystals under a variety of pressure, temperature, and loading rates highly relevant to the Air Force applications. While previous work on data-driven models has often focused on complete substitutions of constitutive laws with a data-driven paradigm, I intend to seek the best option representing the hierarchy of material responses, while implementing adversarial attacks to determine hidden weaknesses of existing polycrystal plasticity models as well as the one generated from the ML approaches. I will make use of a collocation Fast Fourier Transformation (FFT) solver to speed up the generations of the material database, digesting microstructural data via descriptors in the non-Euclidean space, Graph-based knowledge abstraction, and adversarial attack.
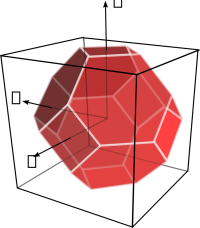