This position is associated with a new research project co-funded by the Earth Institute Frontiers seed funding and the UN Development Programme in Guinea. Its goal is to develop remote sensing technologies to track environmental impact from bauxite mining in rural communities and to help establish protocols for their use. We seek a research assistant who can (1) develop image classification to be applied to satellite imagery to determine extent and abundance of bauxite dust within specific communities in the Boké region (2) develop methods to make classified images available for download to smartphones in the field and (3) adapt existing application technologies to allow users in the field to upload field photographs and locations of potential impacts they identify. The resulting map would represent a composite of satellite imaged areas of impact verified in the field and documentation of other areas of impact not visible by satellite but already identified in the field.
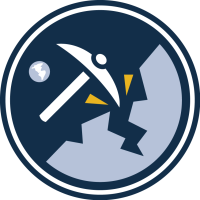