Galaxies in our universe form hierarchically, continuously merging and absorbing smaller galaxies over cosmic time. In this project we aim to identify the most important features of, as well as generate efficient new features from, the merger histories of galaxies. Namely, features that predict (or physically speaking, determine) the properties of galaxies, e.g. their shape or color. This will be done using the results from a large cosmological simulation, IllustrisTNG (www.tng-project.org). We will begin with identifying ways to represent the rich information in the merger history. We will then compare various ML methods oriented towards feature selection or importance analysis: random forests or gradient boosted trees, L1SVM, neural networks (through analysis of e.g. saliency maps). More advanced models can also be applied, such as neural network models designed for feature selection. Finally, we wish to apply / develop methods that can build ‘interpretable’ new features by constructing them as algebraic formulas from original input features (inspired by e.g. https://science.sciencemag.org/content/324/5923/81). The overarching goal is to understand better what in the merger history is most crucial in determining a galaxy’s present-day properties, an answer to which can be widely applicable to problems in galaxy formation.
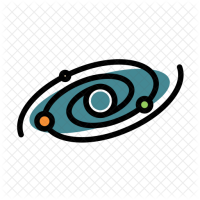