Since the industrial revolution the atmosphere has continued to warm due to an accumulation of carbon. Terrestrial ecosystems play a crucial role in quelling the effects of climate change by storing atmospheric carbon in biomass and in the soils. In order to inform carbon reduction policy an accurate quantification of land-air carbon fluxes is necessary. To quantify the terrestrial CO2 exchange, direct monitoring of surface carbon fluxes at few locations across the globe provide valuable observations. However, this data is sparse in both space and time, and is thus unable to provide an estimate of the global spatiotemporal changes, as well as rare extreme conditions (droughts, heatwaves). In this project we will first use synthetic data and sample CO2 fluxes from a simulation of the Earth system at observation locations and then use various machine learning algorithms (neural networks, boosting, GANs) to reconstruct the model’s CO2 flux at all locations. We will then evaluate the performance of each method using a suite of regression metrics. Finally, time permitting, we will apply these methods to real observations. This project provides a way of evaluating the performance of machine learning methods as they are used in Earth science.
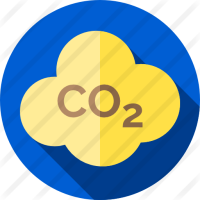