A major challenge to implementing precision medicine arises from patients who share a clinical diagnosis but have different biological causes of disease. Disease subtypes that arise from obscure etiological heterogeneity create inefficiencies in healthcare and attenuate power in clinical trials and research studies. The ability to stratify patients into biologically homogenous subgroups improves the potential for translational research by allowing us to design more powerful studies.
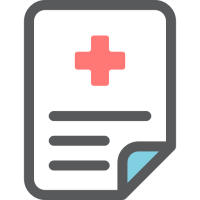