Atherosclerosis—a chronic inflammatory disease of the artery wall—is the underlying cause of human coronary heart diseases. Cells within atherosclerotic lesions are heterogeneous and dynamic. Their pathological features have been characterized by histology and flow cytometry and more recently, by bulk-tissue omics profiling. Despite this progress, our knowledge of cell types and their roles in atherogenesis remains incomplete because of masking of differences across cells when using genomic measurement at bulk level. Single-cell RNA sequencing (scRNA-seq) has catalyzed a revolution in understanding of cellular heterogeneity in organ systems and diseases. This project applies scRNA-seq to define the genetic influences on cell subpopulations and functions in atherosclerotic lesion of transgenic mice for candidate risk genes of human coronary heart diseases as inspired by human genomic discoveries. The students involved in this project are expected to work on: (1) analysis of scRNA-seq data using R/Bioconductor packages; (2) Interpretation of the data using pathway and network analysis. Some relevant workflows are available through the “Resources” page of our lab website at https://hanruizhang.github.io/zhanglab/.
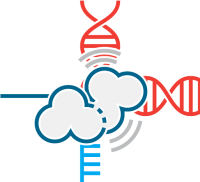