The ocean has absorbed the equivalent of 41% of industrial-age fossil carbon emissions. In the future, this rate of this ocean carbon sink will determine how much of mankind’s emissions remain in the atmosphere and drive climate change. To quantify the ocean carbon sink, surface ocean pCO2 must be known, but cannot be measured from satellite; instead it requires direct sampling across the vast and dangerous oceans. Thus, there will never be enough observations to directly estimate the carbon sink as it evolves. Data science can fill this gap by offering robust approaches to extrapolate from sparse observations to full coverage fields given auxiliary data that can be measured remotely.
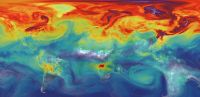