The CONCERN project aims to develop models and tools to quantify clinician concern about patient deterioration in the inpatient setting that can be used in early warning scores. We have discovered and validated several measurable ways within the Electronic Health Record (EHR) to measure clinician concern and have demonstrated that our approach identified patients at risk of deterioration earlier than other methods, which focus only on physiological data. One of our approaches is leveraging documentation of certain concepts within narrative text in nursing notes that are consistent with concern about a patient. However, this narrative free text is not easily accessible - it is often mixed together with structured or templated text and varies over note types. The steps to be performed are
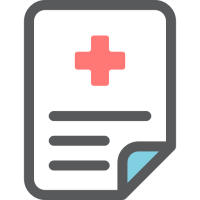