Humanity thrives along major rivers – this is as true now as it was ages ago. Our dependence on rivers for agriculture and electricity, as well as the need to control its flow because of our proximity, has resulted in dramatic changes to the nature of the rivers. What were once great perennial rivers are now mere trickles during the summer months. This puts the livelihood of many people, especially poor farmers, in jeopardy. How can we monitor and document changes to the flow through rivers over time? Since river gauge measurements are rare or non-existent, any way in which we can use freely available satellite imagery (Landsat, Sentinel) to determine the changes in flow patterns of rivers over time would be extremely useful. One such tool is Rivamap – it uses OpenCV to analyze satellite imagery to extract information about rivers, especially for large rivers. What about smaller ones – it does not seem to work as well. In this project, the student(s) will have to develop machine-learning based methods (or extend the capabilities of Rivamap) to study satellite images to extract information about the path and dimensions of rivers of different flow rates and flow patterns. Comparison with ground-truth data will be needed.
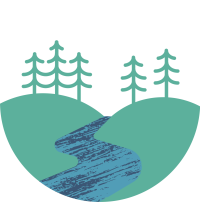