The objective of this project is to construct linkages across disparate public health data systems using machine learning tools and assess them for bias and equitable representation of subpopulations defined by demographic and socioeconomic factors.
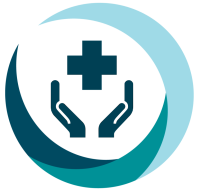
The objective of this project is to construct linkages across disparate public health data systems using machine learning tools and assess them for bias and equitable representation of subpopulations defined by demographic and socioeconomic factors.
This project will be focused on creating a deep learning framework for tracking individual molecules and proteins as they move within a cell under various conditions. Using total internal reflection (TIRF) microscopy, we have accumulated more than 10 million trajectories over dozens of experimental preparations with differences in both the imaging approaches as well as the biological context. In our experiments we have captured particles under a wide variety of conditions including increased protein expression level, and a range of drug concentrations. Our biggest challenge is being able to stably track the movement of a particle as it passes by other particles or groups of particles, and to do this in a way that generalizes over novel conditions. The Data Science Institute Scholar chosen for this project would work with scientists in the Javitch laboratory and others across the Columbia campus to conceive of an approach for efficiently and effectively tracking particles. The resulting work would be of great interest to an increasing number of scientists working in this field who currently rely on methods based on feature engineering that are often inaccurate or inflexible compared to modern deep learning methods.
Big data with temporal dependence brings unique challenges in effective prediction and data analysis. The complex high-dimensional interactions between observations in such data brings unique challenges which standard off-the-shelf machine learning algorithms cannot handle. Even basic tasks of clustering, visualization and identification of recurring patterns are difficult.
The amount of video content that is being distributed over the Internet is increasing. Video providers rely on HTTP adaptive streaming approaches to deliver video clips to users. Complementary to the video provider, the service provider must determine the priority of each network stream. As part of the project, students will explore wireless network assisted strategies for http adaptive streaming by use of TOS/DSCP. This includes using machine-learning tools to analyze network video traffic and the design of reinforcement learning algorithms to improve users' video Quality of Experience.
A central issue facing systems neuroscience is defining the rich naturalistic behavioral repertoire that mice engage in under psychiatrically relevant situations. Recent advances in deep learning (e.g., DeepLabCut) have made frame by frame detailed pose estimation possible. However, this dense behavioral data requires new techniques for defining the ethogram (full description of behavior). To date, researchers have used frequency based time series approaches to tackle this problem, with significant limitations. An alternative approach would be to take advantage of new topology methods (persistent homology and directed algebraic topology) to characterize the shapes formed by mouse limb trajectories. Such an approach would have broad application in systems neuroscience. For this project, the student will use machine learning to label animal body parts, then topology to characterize the ethogram and compare the results to existing approaches.
Many of the cryptocurrency transactions have involved fraudulent activities including ponzi schemes, ransomware as well money-laundering. The objective is to use Graph Machine Learning methods to identify the miscreants on Bitcoin and Etherium Networks. There are many challenges including the amount of data in 100s of Gigabytes, creation and scalability of algorithms.
Under United States securities laws corporations must disclose material risks to their operations. Human rights issues, especially in authoritarian countries, rarely show up in the information that data providers offer to investors, in part due to the risks to those subject to these abuses. The result is a dearth of data on human rights materiality and the tendency of investors to overlook human rights risks of the companies that they finance.