We are requesting a DSI Scholar position for an undergraduate to work with myself and my collaborator Ben Holtzman (Lamont Doherty Earth Observatory, LDEO). We have been collaborating on the development of novel machine learning applications to seismology, specifically unsupervised feature extraction in spectral properties of large numbers of small earthquakes. Our first application was published in Science Advances last year (Holtzman, Pate, Paisley, Waldhauser, Repetto, “Machine learning reveals cyclic changes in seismic source spectra in Geysers geothermal field.” Science Advances 4, eaao2929. doi:10.1126/sciadv.aao2929, 2018). Currently we are building a synthetic dataset to better understand the features that control clustering behavior, and compare different clustering methods.
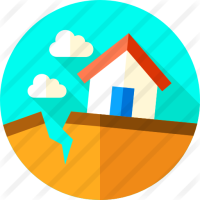