A Fall 2018 internship is available in the Eaton lab to work on the development and application of machine learning approaches to historical evolutionary inference. Research will involve learning to use high performance distributed computing infrastructure, performing population genetic simulations, fitting machine learning models, and writing reproducible shareable code. The ideal candidate will have experience and interest in Python coding and a reasonable understanding of linear algebra.
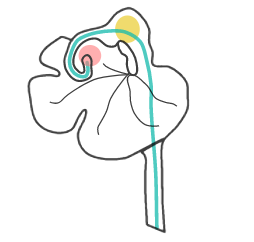