Individuals with schizophrenia shows a wide range of cognitive deficits, and lack in the ability to control the variety of inputs (whether internal or external) to create a unitary “self”. This problem has been often associated with a supramodal attentional deficit, but its causes are yet unknown. In the past twenty years, the quest to identify brain regions or networks showing abnormal functioning in schizophrenia has been unproductive; but one question is left unanswered: is it possible that it is the white matter tracts supporting attention (i.e., the superior longitudinal fasciculi I, II, and III) to be compromised in this psychiatric disorder? The present project will attempt to dissect the fronto-parietal branches with DTI by analyzing over 400 images from the Open Access SchizConnect.Org repository (data already available to the PI). Funds will be used to support the work of a junior or senior-year undergraduate student (possibly from URMs) to conduct the data analysis during the summer of AY2020. Results will be made available with the scientific community and published in open access peer-review journals (e.g., Schizophrenia Research). Co-mentoring of the undergraduate student will be done by Dr. Henrietta Howells (https://scholar.google.it/citations?user=nudmODkAAAAJ&hl=en&oi=ao), opening the student to an international collaboration.
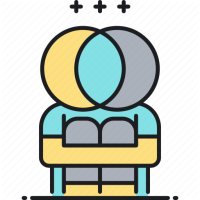