Through ArXivLab we aim to develop the next generation recommender systems for the scientific literature using statistical machine learning approaches. In collaboration with ArXiv we are currently developing a new scholarly literature browser which will be able to extract knowledge implicit in the mathematical and scientific literature, offer advanced mathematical search capabilities and provide personalized recommendations.
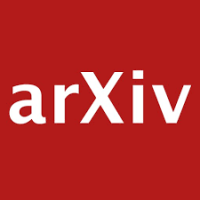