Vehicle-to-Vehicle (V2V) has received increasing attention with the development of autonomous driving technology. It is believed that multi-vehicular and multi-informative algorithm is the direction of the autonomous driving technology. However, the stability and liability of the communication prevents the future from extensively embracing V2V-based transportation. Rigorous test is required before V2V can actually hit the road. Compared with the costly field test, simulation tests are more economical and feasible. To simulate the V2V communication and evaluate the robustness of current V2V-based algorithm, we are therefore developing a simulation platform integrating different commercial software like SUMO, Veins and OMNET++. These software simulate on the actual New York map, and simulate the vehicular communication in different scenarios and platoon configurations. Our next step is to use this platform to test our own V2V-based algorithms. The output of this research will eventually provide an open platform which would automatically evaluate personally designed algorithm with least manual work.
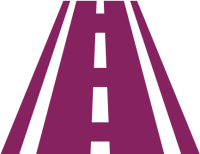