Last year one of my graduate students developed a novel algorithm for detecting “weird” signals in photometric time series, such as those taken by NASA’s Kepler Mission and now TESS. An undergraduate students will work in my team to run the algorithm on TESS data, which is just starting to be released publicly (https://heasarc.gsfc.nasa.gov/docs/tess/status.html). We hope to detect strange signatures, possibly including analogs to Tabby’s Star, interacting binaries and perhaps even technosignatures.
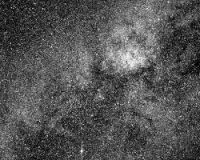