Chronic exposure to arsenic (As) in groundwater is a staggering global public health crisis and yet, we lack a complete understanding of the environmental conditions that govern As mobility and toxicity in groundwater and are unable predict groundwater As concentrations with enough confidence to make effective management decisions. The objective of this project is to identify key hydrologic and biogeochemical variables that control groundwater As concentrations and heterogeneity across spatial scales in Southeast Asia and the USA. We then aim to develop clear mechanistic linkages and high-resolution geospatial information that can be used with machine learning to evaluate and predict groundwater As contamination. This project involves the integration of various types of large datasets from remotely-sensed and field-collected measurements (e.g., surface hydrology and topography, groundwater geochemistry, climate, and population density). We are looking for a student to advance the connections between key environmental variables and groundwater As contamination across scales. The student will receive experience and mentorship in cutting-edge research that crosses interdisciplinary fields, and will have the opportunity to lead their own project and acquire analytical skills using creative measures, which can involve remote sensing, geospatial methods, statistics and graphing, machine learning, and predictive modeling.
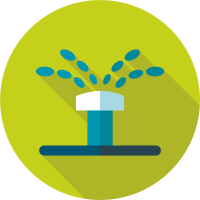