Our goal is to use deep learning networks to understand which neurons in the brain encode fine motor movements in mice. We collected large datasets entailing calcium imaging data of active neurons and high-resolution videos when mice perform motor tasks. We want to use recent advances in deep learning to (1) estimate the poses of mouse body parts at a high spatiotemporal resolution (2) extract behaviorally-relevant information and (3) align them with neural activity data. Behavioral video analysis is made possible by transfer learning, the ability to take a network that was trained on a task with a large supervised dataset and utilize it on a small supervised dataset. This has been used e.g. in a human pose–estimation algorithm called DeeperCut. Recently, such algorithms were tailored for use in the laboratory in a Python-based toolbox known as DeepLabCut, providing a tool for high-throughput behavioral video analysis.
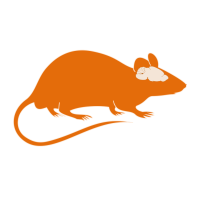