Columbia University Data Science Institute is pleased to announce a new initiative under the Data Science Institute (DSI) Scholars Program: “Data for Good” undergraduate scholars who will work on projects with societal impacts. For the program’s inaugural term, Spring-Summer 2019, we are calling for applications from Columbia and Barnard undergraduate students.
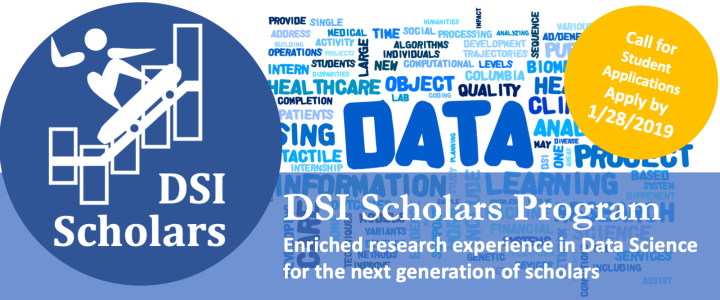
Columbia University Data Science Institute is pleased to announce that the Data Science Institute (DSI) Scholars Program for Spring-Summer 2019 is open for application. The goal of the DSI Scholars Program is to engage Columbia University’s undergraduate and master’s students in data science research with Columbia faculty through a research internship. The program connects students with research projects across Columbia and provides student researchers with an additional learning experience and networking opportunities. Through unique enrichment activities, this program aims to foster a learning and collaborative community in data science at Columbia.
Our lives are heavily reliant on Internet-connected devices and services. However, to deliver the desired user experience over the Internet, network operators need to detect and diagnose various network events (e.g., disruption, outage, misconfiguration, etc.) as well as resolve them in real-time. We have developed an Internet-wide measurement infrastructure that collects performance metrics (e.g., latency, jitter, throughput, packet loss rate, signal strength, etc.) from vantage points deployed by real users (mobile phones, WiFi access points, etc.) at regular intervals.
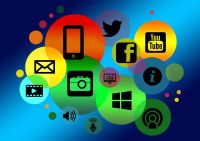
We are interested in investigating how deaths and hospitalizations resulting from opioid overdoses cluster across space and time in the US. This analysis will be conducted with the aid of two comprehensive databases: 1) detailed mortality data across the US; and 2) a stratified sample of all hospitalizations in the US, which can be subset to select for opioid overdoses. Analyses will be extended to drug type (prescription drugs, fentanyl etc.) and subject demographics (age, race, etc.). We have previously conducted similar cluster analysis for other health phenomena.
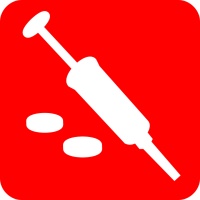
Through ArXivLab we aim to develop the next generation recommender systems for the scientific literature using statistical machine learning approaches. In collaboration with ArXiv we are currently developing a new scholarly literature browser which will be able to extract knowledge implicit in the mathematical and scientific literature, offer advanced mathematical search capabilities and provide personalized recommendations.
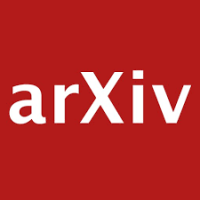
Defective efferocytosis, the phagocytic clearance of apoptotic cells, by macrophages is the cause of many human diseases including tumor, autoimmune diseases and atherosclerosis. Enhancing efferocytosis has potential therapeutic benefits. Many key regulators of efferocytosis have been identified, but a systematic approach to map regulators of efferocytosis in an unbiased manner on a genome-wide scale is missing. This project applies innovative genome-wide CRISPR screen to discover novel regulators of macrophage efferocytosis.
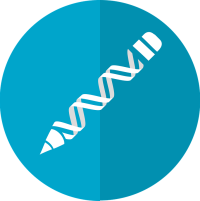
The visual cortex has a distinctive deep hierarchical organization as a result of ontogenetic and phylogenetic optimization. It is unclear what the factors are that shape this particular hierarchical organization. One factor is the compositional and hierarchical nature of our world’s appearance, which may be optimally processed by a hierarchical visual system. Another factor is the need for space and energy efficiency, which constrains the number of neurons and connections. The project will employ computational modeling to understand the contribution of these constraints to shaping the combination of breadth, depth, and skipping connections employed by primate visual cortex.
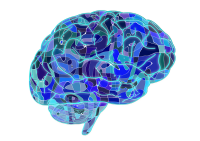