When a colorectal cancer has grown through the wall of the colon or rectum and into other adjacent tissues or organs, it is identified as a T4 primary tumor. If there is no evidence of distant metastasis then it is labeled a locally advanced tumor. Such locally advanced tumors account for approximately 5-15 % of new colorectal cancers. Surgery remains the principal treatment modality for patients with locally advanced colorectal cancer. Studies have demonstrated planned en bloc or multivisceral resections rather than intraoperative assessment of margins more likely results in R0 resections leading to better local control and long-term survival. However, the decision-making for a surgeon confronting a T4 colorectal cancer is challenging because surgery related mortality rates after multivisceral resections are reported up to 12%.
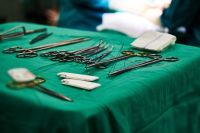